Technical Overview
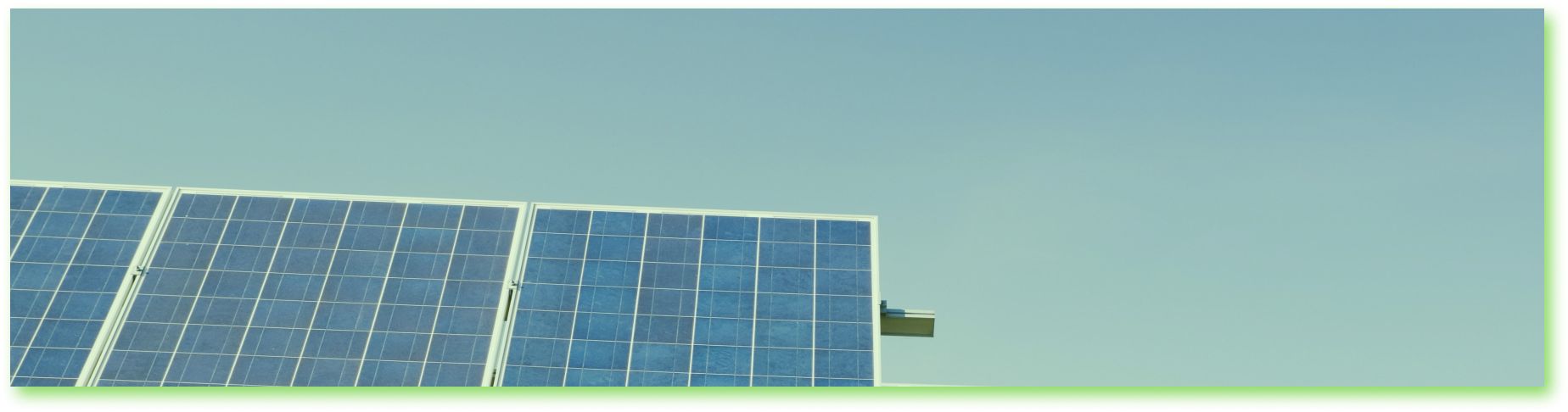
The Solar Planner is a product of rigorous research and development by AI Energy (ApS), a Denmark-based startup co-founded by a team of engineers with PhDs specializing in energy technology and statistics. This document provides a detailed technical breakdown of the features and methodologies employed by our Solar Planner.
ROOFTOP RECOGNITION
Image Processing:
Advanced proprietary algorithms are employed to analyze aerial imagery (Lidar) and detect rooftops and their technical specifications.
Accuracy Metrics:
Tests performed in Denmark indicate a 95% rooftop detection accuracy. Detection rates may fluctuate slightly based on regional topographical variations.
Uncertainty:
Rooftop slope and orientation come with an approximate 2 degree uncertainty, while area estimation holds a 10% uncertainty. Rooftop windows parralel to a rooftop side may not be discernible.
Panel Configuration:
Approximately 70% of each rooftop side is allowed to be populated with panels in the automated panel placement, considering installation ease and area estimation uncertainty.
Shading Analysis:
Shading analysis from nearby buildings and vegetation is calculated hourly-wise, based on the sun’s position. Shading from possibly tall mountains in a region are not taken into account. In Step 1, the shading percentage presents the share of hours in a year when there is shading. However, in Step 3, the shading value presents the solar generation reduction (in energy terms) due to the shading compared to the ideal case with no shading at all.
SOLAR PRODUCTION
Climate models:
Based on calculated rooftop slope, orientation, and location, hourly solar simulation is done based on SARAH2 climate model. Solar production is simulated based on monocrystalline PV modules with string inverters and microinverters, based on the users's choice.
The solar generation is simulated based on a typical year which is sampled from the 10 years of data for a particular location.
OPTIMIZATION PROCEDURES
Mathematical Framework:
Mathematically speaking, optimization that is solved is Mixed Integer Linear Program (MILP) that has the objective of maximizing Net Present Value (minimizing payback period). Specific taxes, regulations and prices are implemented for each country that we support, creating high precision in the economic calculation. As soon as the regulation, distribution fees, taxes, and prices change, this is loaded into the Solar Planner.
Temporal resolution:
Optimization is carried out hourly, and detailed consumption profiles, solar generation, and battery operation are optimized for each hour of the year. Hourly consumption profiles are estimated based on an average household, as well as on information on heat pumps and electric vehicles. If the users has the hourly consumption data, this can be uploaded directly in order to increase the precision of the calculation.
Price Integration:
Hourly pricing data, where applicable, is sourced from day ahead markets.
LIFESPAN PROJECTIONS
Component lifetime:
Solar modules possess a 20-year longevity, whereas the expected operational span for inverters and batteries is 15 years. This is taken into account as a lifetime for calculating economic indicators. A yearly degradation rate of 2% has been assumed for solar panels.
BATTERY SPECIFICATIONS
Configuration and Capacity:
Lithium batteries are incorporated in the model. Their charge/discharge capacity (kW) is constrained to 50% of the total battery capacity (kWh), with a discharge depth capped at 90%.
EMISSION CALCULATIONS
Emission Analytics:
CO2 emissions savings are calculated hourly, based on the specific electricity generation mix in each country/area. The CO2 emissions are calculated based on the weighted average carbon emissions of all production plants in each hour.
ANCILLARY MODULES: ELECTRIC VEHICLES & HEAT PUMPS
Data Enrichment:
Introducing optional details related to electric vehicles and heat pumps augments the precision of the Solar Planner’s outputs.
Financial Scope:
All the economic indicators (KPIs) are calculated solely based on the solar panels and batteries setup, and the investment in EV chargers and a heat pump is not taken into account.
For Inquiries
For further technical clarifications or feedback, reach out to us at contact@solarplanner.eu.